Be part of the solution and make a difference
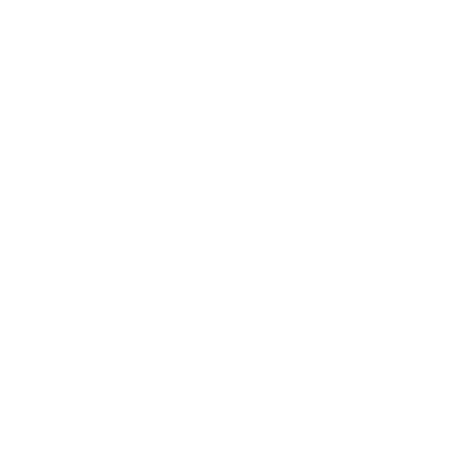
Knowledge Bank - Sign-in
The PAPAA Knowledge Bank is a comprehensive collection of enhanced information, images, educational support programmes and other content. To get FREE access sign-up, it's easy, just provide your name, a valid email and a password.